Ultrafast 3D ultrasound (US) imaging is being developed in research laboratories. However, its clinical application is hindered by insufficient image quality and complex hardware solutions. One of the objectives of the DELTA ANR project is to address this limitation by proposing various signal and image processing methods for morphological 3D US volumetric imaging in cardiology. This first step is required to propose advances in functional and local tissue characterization during strokes. Indeed, during ischemia, the tissue structure undergoes various changes, such as edema and reperfusion, and patient management remains under study. Thus, obtaining local tissue markers related to the local cardiac tissue is crucial for improving patient management and assessing treatment success. High-quality real-time 3D imaging is necessary to allow practitioners to visualize the heart and perform local measurements.
The goal of this internship is to use Deep learning algorithms to reconstruct a high-resolution 3D volume from a limited number of low-resolution volumes. This learning process will involve synthetic and experimental acquisitions. Simulations will be performed using the MUST toolbox [1], and the lab's previous expertise in 2D cardiac imaging will be a starting point for future networks [2]. Moreover, 3D model of the heart exists, and ultrasound images could be generated from this temporal model. Then, using two different 3D experimental laboratory systems, the strategy will be evaluated on the real volumetric dataset. Depending on the project advancement, the acquisition of a temporal volumetric dataset will also be conducted on a rotary disk to assess the potential of the US volumes to perform motion estimation. Two kind of volumetric imaging could be targeted: a complete volume generation or a tri-plane one, which requires less computation load and could lead to easiest real-time rendering.
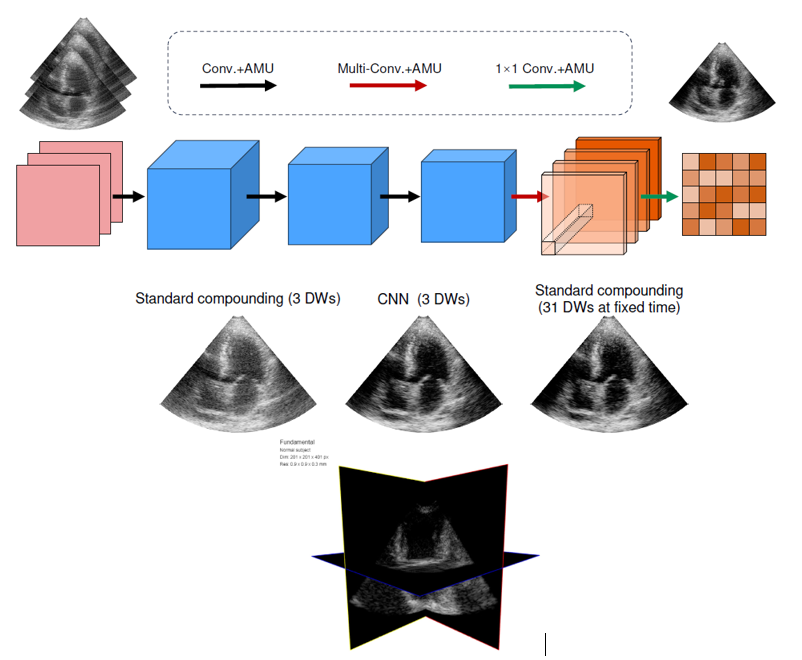
Previous pipeline used for 2D cardiac image beamforming. Similar approach could be used for generation of high-quality 3D cardiac imaging [2]. Example of tri-plan cardiac image is shown on the figure above.
In summary, the objectives of the proposed internship are:
Propose different US volume reconstruction strategies to create the training dataset for high-resolution volumetric US imaging.
Starting from a previous network developed in 2D, propose and create a new network for 3D imaging using low-resolution US volumes.
Validate the proposed network on experimental imaging and evaluate the temporal consistency of the network.
Depending on the project's advancement, the inference could be implemented on the experimental system of the lab.
General information
Profile: Student from a top engineering school or research master's degree (generalist or EEA profile)
Technical skills: image and signal processing and/or machine learning
Soft skills: Versatility and curiosity, ability to work independently, initiative, good written and oral communication skills.
Start and duration of placement: February/March 2025 for 6 months.
Workplace: Creatis, la Doua campus.
Depending on the developments and results of this initial work, a PhD may be envisaged in the DELTA ANR project.
How to apply
Send CV + cover letter + M1/M2 or engineering school transcripts to:
- François Varray, Associate Professor, francois.varray@creatis.insa-lyon.fr
- Fabien Millioz, Associate Professor, fabien.millioz@univ-lyon1.fr
- Valentine Wargnier-Dauchelle, Associate Professor, Valentine.Wargnier@creatis.insa-lyon.fr
References
[1] Garcia D, Varray F. SIMUS3: an open-source simulator for 3-D ultrasound imaging. Comput Methods Programs Biomed, 2024;250:108169.
[2] J. Lu, F. Millioz, D. Garcia, S. Salles, D. Ye, and D. Friboulet, “Complex convolutional neural networks for ultrafast ultrasound imaging reconstruction from in-phase/quadrature signal,” IEEE Transactions on Ultrasonics, Ferroelectrics, and Frequency Control, vol. 69, no. 2, pp. 592–603, 2022.